GRAIL 2017 Proceedings
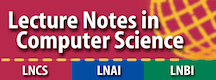
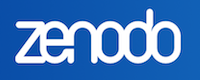
The complete proceedings are available in two formats:
- as a Springer LNCS Book
- and an open access version in Zenodo
Open Reviews: Index
Uncertainty Estimation in Vascular Networks
Markus Rempfler, Bjoern Andres, Bjoern H. Menze
Extraction of Airways with Probabilistic State-space Models and Bayesian Smoothing
Raghavendra Selvan, Jens Petersen, Jesper Pedersen, Marleen de Bruijne
Classifying phenotypes based on the community structure of human brain networks
Anvar Kurmukov, Marina Ananyeva, Yulia Dodonova, Joshua Faskowitz, Boris Gutman, Neda Jahanshad, Paul Thompson, Leonid Zhukov
Autism Spectrum Disorder Diagnosis Using Sparse Graph Embedding of Morphological Brain Networks
Carrie Morris, Islem Rekik
Topology of surface displacement shape feature in subcortical structures
Amanmeet Garg, Donghuan Lu, Karteek Popuri, Mirza Faisal Beg
Detection and Localization of Landmarks in the Lower Extremities Using an Automatically Learned Conditional Random Field
Alexander O Mader, Cristian Lorenz, Martin Bergtholdt, Jens von Berg, Hauke Schramm, Jan Modersitzki, Carsten Meyer
Graph Geodesics to Find Progressively Similar Skin Lesion Images
Jeremy Kawahara, Kathleen P. Moriarty, Ghassan Hamarneh
Uncertainty Estimation in Vascular Networks
Markus Rempfler, Bjoern Andres, Bjoern H. Menze
REVIEWER #1
1. Level of expertise
- Knowledgeable
2. Recommendation
- Accept
3. Summary of the paper
- The authors propose to extend an existing approach for reconstructing the vascular network in fundus photographs by incorporating a method for quantifying the uncertainty of the probabilistic model. To this end, they evaluate two different sampling approaches, a perturbation sampler and a Gibbs sampler, both of them based on previous studies. A series of experiments on a data set of fundus images is performed, showing that the Gibbs sampler is able to achieve a smaller mean absolute approximation error than the perturbation sampler, although at the cost of higher variance when fewer samples are taken. Qualitative results show that the perturbation sampler, however, yields lower level of uncertainty on obvious vessel segments compared with the Gibbs sampler, with higher levels reported at the end of each vascular segment or in branching/crossing points.
4. Strengths
- The extension proposed by the authors is extremely valuable as, in principle, it would allow the users to threshold the output mappings to decide which levels of uncertainty can be clinically tolerated and incorporated in the binary network.
The provided references and the mathematical background given in the draft are sufficient to understand the nature of the problem and how it is tackled with the Gibbs and the perturbation samplers.
5. Shortcomings
- The paper is not properly organized, as details about key elements of the vascular network such as the nodes and edges of the graphs are given in the experiments section rather than in the Background or Introduction sections.
Although authors indicate that the Gibbs sampler requires to take a larger amount of samples to achieve better error rates than the perturbation sampler, no insights about how it affects the computational efficiency of the method are provided. Thus, the reader cannot decide which of the models is better in terms of this feature.
No hypothesis tests are provided to estimate if the difference in the results are statistically significant.
6. Constructive feedback
- - The explanation about the candidate graph and how it is obtained should be moved at the very beginning of the Background section. Otherwise, the reader has to go to the provided references or to Section 4 to finally understand the graph structure.
- Fig. 3 shows that the Gibbs sampler produces higher uncertainty levels throughout the entire vascular structure. As a consequence, I think that thresholding this graph will result in isolated segments that will not represent the vascular network properly. Am I wrong with this assumption? In case it is valid, please clarify in the main text how do think that this issue could affect the final results.
- Authors still have room in the paper to incorporate an additional figure comparing the computational efficiency of each sampling approach. It would also be interesting to compare the decrease in the mean absolute approximation error for each of the models within the same figure, to see if they are representative enough.
Some other minor corrections should also be addressed:
* Abstract. "Reconstructing vascular networks is a challenging task in medical image processing THAT OFTEN HAS TO DEAL with large variations in vessel shape and image quality". Not often but always. I suggest the author(s) to modify this sentence to: "Reconstructing vascular networks is a challenging task in medical image processing AS AUTOMATED METHODS HAVE TO DEAL with large variations in vessel shape and image quality".
* References should be ordered by usage. (e.g., first reference is 10 and should be numbered 1).
* Citation needed at the end of this sentence: "Analysing vascular graphs is expected to give insights into various biological properties, e.g. the relation between vascular remodeling processes and neurological diseases or pharmaceutical treatments".
* There is a repeated AS in this sentence in Section 2 (Background): "vessel network reconstruction pose the problem AS AS MAP inference in a (constrained) probabilistic model".
REVIEWER #2
1. Level of expertise
- Expert
2. Recommendation
- Accept
3. Summary of the paper
- Two different methods (perturbation and Gibbs sampling) for quantification of uncertainties in vascular graphs are given. These techniques are useful to allow user have an evaluation of qualities of MAP segmentations representing vascular graphs for a better averaging and inference of overall underling structures.
4. Strengths
- The paper is well written and discusses an interesting problem. The theory is sound.
5. Shortcomings
- The experimental section could be showing the usefulness of the paper and validation could be based on a synthetic ground truth data currently missing.
Mathematical notions could be better, for instance there is no explicit definitions for x_C and \mathcal{C}(G).
6. Constructive feedback
- What is meant by higher level events? this should be any configuration of labels on three edges connected to a node i guess. But these can be better defined.
Please mention how long does it take to check for feasible x\in\Omega. It should be a length process.
Please mention a reference on Gumbel distribution, why such a complex distribution is needed here for sampling?
My understanding is that you marginalize the model parameters here \Theta, when computing the posteriors. If this is true please mention it explicitly.
REVIEWER #3
1. Level of expertise
- Knowledgeable
2. Recommendation
- Accept
3. Summary of the paper
- This paper evaluates practically efficient methods for obtaining uncertainty estimates in the context of vascular network reconstruction. Two sampling based approaches are evaluated and compared on their strengths and weaknesses.
4. Strengths
- The review of relevant work on related problems is thorough. The experiments compare to a reasonable ground truth obtained by brute force. The images shown are also very illustrative.
5. Shortcomings
- None that I could see
6. Constructive feedback
- I am not too familiar with the vascular network reconstruction but it would be interesting to see if the proposed methods can improve performance on the downstream quantitative evaluation
Since the experiments are on smaller graphs it would be interesting to know more about convergence properties as a function of the graph size
Extraction of Airways with Probabilistic State-space Models and Bayesian Smoothing
Raghavendra Selvan, Jens Petersen, Jesper Pedersen, Marleen de Bruijne
REVIEWER #1
1. Level of expertise
- Knowledgeable
2. Recommendation
- Accept
3. Summary of the paper
- The submission proposes a new method for the automatic segmentation of airways from CT chest data.
4. Strengths
- This is a really interesting paper and nice to read. The method is nicely derived and the equations stated. Having a measure of uncertainty could be interesting for the translation to the clinic.
5. Shortcomings
- Error in Eq. 5. Vector x_k is not 7D
Why does the noise q only concern direction and radius?
Eq (19) doesn’t “apply a threshold”, as written in the text
Threshold \mu_c is set to 2.0, but defined based on the trace in Eq (19)
Only comparison to region growing. Consider adding comparisons to state-of-the-art methods.
REVIEWER #2
1. Level of expertise
- Expert
2. Recommendation
- Accept
3. Summary of the paper
- A method similar to particle filter based methods is proposed for tracking branches, with the key difference that can track branches starting from multiple seed points. Transition from one tracking step to another within a branch is modeled using a process model and measurements are modeled with a measurement model. Using Bayesian smoothing a forward backward recursion is used for the estimation of the posterior i.e. the estimation of the states of branches. The tree of most likely branches is then created by removing the false positive branches, using the posterior estimated in Bayesian smoothing. Average of the diagonal elements of the covariance matrices estimated for the posterior distributions of the branch steps.
The method is applied to the problem of tracking airways in lung CT images. 16 images were randomly chosen out of a dataset containing 32 CT images. Segmentation results were compared to the region growing method and outperforms it in terms of the distance of segmented center lines to the reference center lines
4. Strengths
- The paper is presenting a novel method for tracking branches in images. The proposed outperforms the region growing in segmentation of lung CT images. The language is precise and the paper is very well written.
5. Shortcomings
- The start of algorithm using several seed points can cause issues if those seed points aren't properly located. Too much details are given in the abstract.
6. Constructive feedback
- Eq. 5 needs to be corrected before issuance.
REVIEWER #3
1. Level of expertise
- Knowledgeable
2. Recommendation
- Accept
3. Summary of the paper
- The paper proposed an application of Bayesian smoothing method for the segmentation of tree-like structures in medical images like vessels, neurons, and airways. Bayesian smoothing estimates the state of a dynamic system which is indirectly observed through noisy measurements. In proposed method, each branch of the tree like structure is formulated as a sequence of states (position, radius, direction), which are estimated as Gaussian density process by observing measurements. The measurements here are the location and radius of the blobs detected on different scales, on a probability image computed using k-Nearest Neighbour voxel classifier. The Bayesian smoothing is performed using a two-filter RTS smoother. The first filter is a Kalman filter, with a prediction and update step. In the prediction step, it estimates the mean and covariance of Gaussian density of current state given the previous state. In update step, it used the predicted mean and covariance to obtain the posterior density estimates of the system. The second filter is a backward smoothing filter, which computes mean and covariance conditioned on the posterior estimates of all the states in a branch. A threshold on total variance of all the states in a branch, is used to qualify the branch as a true positive.
4. Strengths
- see comments
5. Shortcomings
- see comments
6. Constructive feedback
- • The proposed method is a type of centerline tracking algorithms. To ensure the final states of the branch are in the center of the vessel or neuron or airway, predicted positions/states should be re-centered.
• An analysis of how to calculate the radius of the segmented tube-like structure is missing.
• The success of the method heavily depends on seed point initialization. If initial seeds are missing, there is no way of segmenting that branch.
• In case of occlusion or low-level noise, the model may suffer from premature stopping. The resulting branches will be segmented into multiple pieces. The small connecting pieces may get removed during thresholding, leading to missing segmentation.
• Multiple iterations of the current process may help in recovering missing pieces.
• The thresholding applied to identify true branches, may result in removal of actual branches as evident in Fig 3.
• The proposed method outputs a tree-structure where the different branches are disconnected. It limits the application of the method in cases where location of branching points is important.
• The quantitative comparison of proposed method with other known methods is missing.
• A more extensive experimentation is required to validate the applicability of the method.
• The paper is very well written and the references are adequate.
Classifying phenotypes based on the community structure of human brain networks
Anvar Kurmukov, Marina Ananyeva, Yulia Dodonova, Joshua Faskowitz, Boris Gutman, Neda Jahanshad, Paul Thompson, Leonid Zhukov
REVIEWER #1
1. Level of expertise
- Expert
2. Recommendation
- Accept
3. Summary of the paper
- The paper is proposing a pipeline for the classification of brain disorders (or so-called phenotypes) based on the community structure of the corresponding brain connectivity networks. Following the construction of a structural connectivity network, the proposed pipeline involves detecting the communities within the network, either overlapping or non-overlapping, estimating the distance between the community structures of network pairs and feeding those to a kernel-based classifier for the final classification step. Results of the experiments that test this hypothesis are presented by means of AUC, including different community detection algorithms and distance metrics.
4. Strengths
- This paper is proposing an interesting methodology that attempts to predict disease phenotypes based on the community structure of the subjects' structural brain networks. It is nicely written and provides detailed information about the proposed pipeline. The method is tested on the ADNI dataset and yields superior results compared to l2-norm distance between the original networks for the task of AD vs NC classification. The pipeline could potentially be applied on difference classification or regression problems.
5. Shortcomings
- The authors do not specify why they chose the specific database. Is it a proof of concept or it's because of evidence that structural connectivity is modified in Alzheimer's disease? The experimental setup is a bit vague. How are the optimal parameters for the classifier selected? Is it a grid search in a cross-validation setting? What are the optimal parameters used in the end? This is not mentioned explicitly. It is also unclear whether reported results correspond to average AUC for the 10-fold cross-validation or the 50 random splits.
6. Constructive feedback
- - Add some relevant literature about Alzheimer's disease and studies identifying links to brain connectivity.
- Try replacing "anatomical brain networks" with "structural brain networks".
- "shifts in brain anatomy" does not seem very suitable to describe disruptions to the network structure or anatomical changes.
- Replace "a recent decade".
- Change "triangle mesh" to "triangular mesh".
- Fig 2 is not too informative; maybe worth adding the corresponding connectivity matrix or network.
- Formulas for AMI and NMI should ideally be provided (since there is more space).
- “Human brain networks show modular structure which arises based on the entire system of connections between cortical brain regions. Systematic shifts in connectivity patterns..” needs rephrasing and a better description of what purpose the modular structure in the brain serves.
REVIEWER #2
1. Level of expertise
- Knowledgeable
2. Recommendation
- Reject
3. Summary of the paper
- The authors propose to perform community detection using NMF on the tractography connectivity for the ADNI data.
4. Strengths
- The community hypothesis is worth pursuing.
5. Shortcomings
- * contribution of the paper is not clear.
* There are lots of work in this field even in the MICCAI community. The literature review is not enough. The closest work to that of authors is: "Dominant component analysis of electrophysiological connectivity networks" which is not cited.
* It is well explained why low rank factorization of the adjacency matrix results in a community detection.
* The kernel in eq4 is not necessarily positive definite and there is not a discussion about it.
* I am not sure how one can understand the figure 3
REVIEWER #3
1. Level of expertise
- Expert
2. Recommendation
- Accept
3. Summary of the paper
- The paper compares the community identification in networks based on either non-overlapping communities and overlapping communities. It evaluates how features generated from this community structure are able to classify control subjects and AD patients.
4. Strengths
- Quantitatively evaluating how overlapping communities can be used to capture brain network structure is valuable, and the authors propose interesting alternatives.
5. Shortcomings
- In the current form the feature extraction should be clarified.
At points it is not clear up to which point the overlapping information is actually used for analysis or classification, and at which point (if at all) it is reduced back to a non-overlapping membership assignment.
6. Constructive feedback
- Please clarify (beyond a citation) why overlapping communities are a more powerful descriptipon of the observed data.
Sec.2.2.: please clarify how NMI can be used to evaluate the similarity of overlapping community assignments? The current formulation seems to suggest that the overlapping assignment is binarized to a non-overlapping assignment before evaluating the measure. This would defeat the purpose of the paper, right?
Please be more specific in the description of the features you use for classificaiton. This is hard to follow in the current version.
FIg. 3: can you visualized the multiple memberships? This would be very informative, since it is a central point of the paper.
Can you give an intuition how the mutual information works on the community assignments without generating an explicit matching between corresponding communities?
Autism Spectrum Disorder Diagnosis Using Sparse Graph Embedding of Morphological Brain Networks
Carrie Morris, Islem Rekik
REVIEWER #1
1. Level of expertise
- Expert
2. Recommendation
- Accept
3. Summary of the paper
- The paper is proposing a framework that employs sparse graph embedding of connectomic data, while focusing on morphological brain networks and a high-order representation of those. Following the estimation of multiple morphological brain networks for each subject, a high-order morphological network is constructed that combines connectivity information from the individual networks. A similarity matrix is, subsequently, learnt with sparse graph embedding. A low-dimensional data embedding allows to obtain feature representations for all subjects that can be fed to a linear SVM classifier. The potential of the method is explored on the ABIDE database containing 102 subjects.
4. Strengths
- The methodology proposed in this paper is interesting and is focusing on the relatively unexplored morphological brain networks. The framework accommodates multiple morphological networks allowing for the potential integration of additional modalities in the future. The evaluation experiments compare between different feature vector representations and dimensionality reduction techniques and provide insight into how these different components influence the final ASD vs NC classification results.
5. Shortcomings
- The dataset is imbalanced, so chance level accuracy is approx 60% rendering results not too impressive (probably sensitivity and specificity results are more convincing). It is also unclear why the connectomic data lie in different manifolds and how the number and dimensionality of the manifolds is defined. Selection of the optimisation parameters also needs to be justified, as well as the parameter grid that is explored for SGE and LLE.
Additionally, the ABIDE database provides imaging data for ~1000 subjects and it is unclear why authors choose only 104. What do the presented results correspond to? Cross-validation and, if so, with how many folds? These need to be clarified.
6. Constructive feedback
- - “complex pattern of cognitive impairments” should be rephrased
- “largely outperformed several state-of-the-art methods” sounds like an overstatement
- not sure whether the “clustering coefficient feature vector” is the best way in terms of network measures to summarise the HON; perhaps connection strength could lead to more discriminative features
- the definition of \hat{D}_i seems to allow negative distances; is that a typo?
- not sure why negative and positive weights w_{ij} lead to equivalent similarities (since the similarity matrix is defined using the absolute values of the weights)
- Be careful with N/A values in Bibliography
REVIEWER #2
1. Level of expertise
- Expert
2. Recommendation
- Accept
3. Summary of the paper
- The authors propose a novel ML strategy that uses a graph embedding based representation to discriminate autism patients from healthy controls based on structural brain MRI scans.
4. Strengths
- The machine learning approach and, in particular, the use of high order networks, is innovative. Empirical results on a difficult clinical problem seem promising.
5. Shortcomings
- The high order graphs are not theoretically motivated.
The exploration of several design choices opens up the possibility of over-fitting in the experiments.
Alternative machine learning techniques (in particular based on modern neural networks) are not explored.
A model that used all 4 "views" simultaneously should have been benchmarked too.
Minor point: Eq 1 seems to have typos in it. E.g., optimization is over lambda or alpha? This equation and the corresponding method should have been explained in more detail.
No rationale was presented for setting lambda to 10.
Minor point: the clustering coefficient approach should be spelled out more.
REVIEWER #3
1. Level of expertise
- Knowledgeable
2. Recommendation
- Borderline
3. Summary of the paper
- The paper proposes a graph analysis method for the study of so-called morphological brain networks. These networks capture differences in local morphological features.
4. Strengths
- Capturing relationships of distributed morphological features is an interesting approach.
5. Shortcomings
- Clarity of explanation should be improved, to make the benefit of the higher-order/lower-order approach and the embedding clear. (see feedback for detail)
6. Constructive feedback
- Abstract and introduction can be shortened to make them more concise, and focus on the motivation and contribution of the paper.
Please fix the citations, they contain several incomplete entries
How is the manifold you learn from the vectorized matrices related to a manifold of connectivity matrices - symmetric positive definite matrices?
High-order networks: what is the difference between region-to-region relationships, and relationships between pairs of ROIs. This seems to be the same thing. In the subsequent paragraphs, it remains unclear what the differences is (e.g., the feature extraction seems to be the same). Please clarify.
Is the morphological network capturing the difference of local morphological features for each individual, or is this based on the correlation of these features across the population?
Representing each network by its clustering coefficient seems like an important part of the method. I would move it there, and would also evaluate the impact of this choice.
The results interpretation could be expanded.
REVIEWER #4
1. Level of expertise
- Knowledgeable
2. Recommendation
- Accept
3. Summary of the paper
- This paper proposes to use graph embeddings of morphological networks to improve classification performance in ASD. The authors compare several approaches for doing this on
4. Strengths
- The authors present several approaches and compare them on a challenging dataset. The proposed morphological network modeling appears to give quantitative gains.
5. Shortcomings
- Some of the presentation can be improved. The abstract for example is a bit too dense to give a quick impression. There is similarly quite a few run on sentences in many places and the language can be a bit informal in some places (e.g. “Basically, we”)
Although the comparisons appear fair since the same pre-processing is used for the compared methods, the evaluation on ABIDE doesn't appear to compare to or discuss the state of the art quantitative results reported on this dataset, which may use different pre-processing/parcellation.
The authors should clarify the difference of their work to [8] besides the change in disease.
Topology of surface displacement shape feature in subcortical structures
Amanmeet Garg, Donghuan Lu, Karteek Popuri, Mirza Faisal Beg
REVIEWER #1
- Reviewer did not agree to make his evaluation publicly available.
REVIEWER #2
1. Level of expertise
- Expert
2. Recommendation
- Accept
3. Summary of the paper
- The submission studies the shape of subcortical structures on a population of subjects with Parkinson's disease. Surface displacements are computed for pallidum and caudate. Significant group level differences on the PPMI dataset have been reported.
4. Strengths
- - Shape analysis in Parkinson's disease
- Nice overview of method in Figure 1
- The presentation of the method is clear
5. Shortcomings
- It is mentioned that the interaction between subfields of subcortical structures is studied. Yet, there is no mentioning of subfields in the results section; subfields of caudate or pallidum do not seem to be segmented. While local shape changes in these structures may be associated to changes in subfields, without actually having the subfields, this claim seems too strong. One could also imagine regional changes that do not necessarily have to be linked to subfields.
Report of the computational complexity of the method would be interesting
"The shape of anatomical structures in the brain has shown an adverse influence of neurodegenerative disorders." How can a shape have an adverse influence?
The usage of the word “topology” is sometimes irritating. I understand that the authors refer to network topology. However, when writing “shape topology change with Parkinson’s disease”, the reader may think that about topological changes from sphere to torus.
REVIEWER #3
1. Level of expertise
- Expert
2. Recommendation
- Borderline
3. Summary of the paper
- This paper attempts a new shape analysis method that investigates the inter-regional covariance of shape change, with an application using subcortical structures (caudate/pallidus) in Parkinson's patients.
4. Strengths
- This is an interesting application of the persistent homology ideas, and it could lead to new insights about shape differences between different populations.
5. Shortcomings
- It is frustrating that no other shape analysis method is even mentioned in this paper, let alone a comparison. There is decades worth of literature in this topic, and it is not clear why we need yet another shape analysis method.
I also found it unsatisfying that the different structures are analyzed independently from each other. The main hypothesis of the paper is that it is worthwhile looking at covariation of shape change between different patches of a given surface, which I agree with, but to me it seems just as important that two neighboring structures would be also dependent on each other, e.g. the medial surface of the putamen with the lateral surface of the pallidus.
Is there a way to actually visualize the differences detected by the method? The authors repeatedly claim their goal is to identify inter-regional covariation of shape change, but I find it difficult to do so without actually visualizing what the differences are. The authors also claim as early as in the abstract that this method will be useful in clinical settings, which seems like a complete overreach, especially considering the method in its current form can not predict at the subject level.
It seems the SurfDisp features s_i are just scalar values at each vertex, right? Does that mean the method doesn't take into account any sort of directionality in displacement? How does it differentiate growth from atrophy, or a displacement along the surface normal vs a sliding motion along the tangent?
6. Constructive feedback
- I found the notation difficult to follow, esp the i and k's seem to refer to many different variables (vertices, patches, subjects, thresholds, graph nodes, ...) and they change back and forth between paragraphs.
The authors really need to do a better job of describing the SurfDisp method here, in the interest of making the paper self-contained - there's plenty of space.
Many experimental details are lacking, e.g. how the number of clusters and the clustering scheme is determined for the parcellation step, registration technique used to compute SurfDisp features, etc.
Detection and Localization of Landmarks in the Lower Extremities Using an Automatically Learned Conditional Random Field
Alexander O Mader, Cristian Lorenz, Martin Bergtholdt, Jens von Berg, Hauke Schramm, Jan Modersitzki, Carsten Meyer
REVIEWER #1
1. Level of expertise
- Knowledgeable
2. Recommendation
- Accept
3. Summary of the paper
- The authors propose a random forest / CRF based approach for detecting and localizing landmarks in X-ray scans.
The random forest is used to generate a pseudo probability map to localize the landmarks, while the CRF is applied afterwards to introduce other priors and to correct the output of the classifier.
In particular, the CRF is designed in such a way that the learning process involves both the optimization of the usual energy and the determination of the best possible combination of potentials.
Moreover, authors explicitly adapt their approach such that it is able to deal with images with missing landmarks.
The method is quantitatively and qualitatively evaluated on a private data set of 600 X-ray scans to detect 6 landmarks. Results are promising and outperform the random forest approach without CRF priors and the CRF with a full set of potentials.
4. Strengths
- The CRF part of the method is able to simultaneously optimize the energy and the set of potentials.
The ability of the method to deal with missing landmarks is impressive.
5. Shortcomings
- The feature extraction and the random forest stages have a number of parameters that seems to be adjusted to this specific data set, and no insights about how they were experimentally determined are provided.
The computational efficiency of the method is not discussed in the paper.
6. Constructive feedback
- The L1-norm is used as a regularizer for selecting the best combination of CRF potentials due to its ability to introduce sparsity in the weight vectors. Afterwards, the performance achieved with the resulting potentials is compared with a CRF in which all the weights are set to 1, to justify the removal of the useless potentials. I personally believe that this comparison is not fair, as this weights were not learned from data like the other. If the purpose is to show that some combination of potentials are not useful or degradate the performance of the CRF, authors should optimize the same objective function but replacing the L1-norm by the L2-norm, and use the CRF learned by such an approach in the comparison.
The contribution of removing the useless potentials is only quantified in terms of its improvement in the quality of the results. It would be nice to include also a few sentences and/or a table showing also how the resulting CRF is more computationally efficient that the approach based on the full set of potentials.
Authors should provide insights on how to experimentally adjust the parameters (n=15, minimal distance between peaks = 3 pixels, F=128, A=(351, 351), M=317). This will certainly help the readers to reproduce the paper and/or to apply it to their own data sets.
Some other minor corrections should also be addressed:
* The acronym NMS is not defined.
* Authors mention that they use a "BRIEF-like approach". In that line they should include a reference to Calonder, M., Lepetit, V., Strecha, C., & Fua, P. (2010). BRIEF: Binary robust independent elementary features. Computer Vision–ECCV 2010, 778-792.
* Some sentences in Section 2 start with a numeric citation. It would be nice to use author names followed by the
* Minor proof reading and corrections (in abstract, "does not show all landmarks" should be "does not show all THE landmarks").
REVIEWER #2
1. Level of expertise
- Knowledgeable
2. Recommendation
- Accept
3. Summary of the paper
- This article presents a learning framework to first detect the presence, and then for those landmarks that are present, to locate them, in the lower extremity X-ray images.
4. Strengths
- One main contribution is the design of a pool of potential functions in the CRF to encourage landmarks to co-exist and co-exist with some spatial correlations. Another contribution is to use learning approach to optimize graph parameters instead of using heuristic ones.
Both contributions are legitimate and important.
5. Shortcomings
- Limitations lie in the lack of comparison with existing approaches. Without this context, it is hard to appreciate how accurate or inaccurate the 10mm landmark error reported in this paper. Also, the paper should improve by more explicitly explaining why different potential functions are formulated (clinical motivation), and what are the relationship among those potential functions (ideally figures or sketches).
REVIEWER #3
1. Level of expertise
- Expert
2. Recommendation
- Accept
3. Summary of the paper
- The paper presents a learned CRF approach for landmark localisation on lower extremity x-ray.
4. Strengths
- Nice paper. Very well written with a thorough evaluation. Reasonably large database has been used and results have been analysed systematically providing valuable insights into different components and types of errors that are made by the method.
5. Shortcomings
- A bit weak on the discussion of higher-order CRFs. There is substantial work on efficient higher-order clique optimisation which could be considered for the problem at hand. Due to the small size of the CRF in terms of number of random variables, it should be feasible to employ techniques such as higher-order clique reduction.
6. Constructive feedback
- See above. Future work could explore higher-order cliques to model more complex constraints such as global configuration of landmarks.
Graph Geodesics to Find Progressively Similar Skin Lesion Images
Jeremy Kawahara, Kathleen P. Moriarty, Ghassan Hamarneh
REVIEWER #1
1. Level of expertise
- Knowledgeable
2. Recommendation
- Accept
3. Summary of the paper
- The authors propose to visualise a progression between two skin lesion images through intermediate images by stepping along the geodesic shortest path between the two images. To do this, they create a graph of all the images based on the pairwise dissimilarity between the images' feature representations, which are obtained by a partial forward pass through a pre-trained VGG-16 network.
4. Strengths
- The paper is well written with a convincing motivation for their problem setting. The methodology they present to solve the problem is simple and obtains good results compared to choosing random paths.
5. Shortcomings
- The method seems to rely crucially on the way the graph is constructed, i.e. how the edge weights are calculated and the number of neighbours used to sparsify the graph. Since these factors heavily influence the resulting quality measures, it is unclear how useful and objective the proposed quality measures are. Further comments, mostly regarding the experimental evaluation, are shown below.
6. Constructive feedback
- - The experiments and results section (Sec. 3) could benefit from structuring of the text, as it is difficult to keep track of the different types of experiments peformed.
- The authors perform a synthetic experiment by inserting scaled versions of the same image into the graph, and obtain perfect progression paths including *all* of the inserted synthetic images. However, it is important to note that the pre-trained VGG-16 network used to obtain a feature representation of the images was trained at multiple scales. If the network is somewhat invariant to scale, it would make sense that all synthetic images are similar enough (in features space) to be selected for the path. A discussion of this would be interesting, and possibly a visualisation of the graph, and the synthetic images in the graph.
- Experiment 1.7 performs interpolation in feature space and then chooses the nearest neighbour for each interpolated point to find a path. Quantitatively, the authors state this performs worse than the geodesic path on the graph. This is a surprising finding and a discussion of this would be helpful. Do the authors have an explanation? In theory, if I'm not mistaken, if the feature space was densely (or even regularly) sampled by the data, the results should be the same. Furthermore, the example results in Fig. 3 suggest that the progression of 1.7 is of higher quality than 1.5 (as observed from a layman's perspective).
- The authors do not report any measure of spread for their quantitative results, and it is therefore difficult to assess the consistency and difference in performance between the different tested configurations._
REVIEWER #2
1. Level of expertise
- Expert
2. Recommendation
- Borderline
3. Summary of the paper
- The paper describes the application of a simple graph geodesic / shortest path algorithm to find a transition of 'progressively' similar images in a database of skin lesion images.
In my opinion, the paper addresses an interesting application/problem but has several severe shortcomings and does not provide enough methodological insight to warrant discussion at GRAIL.
4. Strengths
- The use of a p=4 distance, seems to compensate some problems for densely connected kNN-graphs.
The introduced surrogate metrics are useful.
5. Shortcomings
- The authors describe the images by CNN features pre-trained using ImageNet. Strangely, no domain specific training or at least fine-tuning on a skin lesion database, such as the publicly available ISIC (see https://challenge.kitware.com/#challenge/n/ISIC_2017%3A_Skin_Lesion_Analysis_Towards_Melanoma_Detection), is performed. Therefore, no semantic similarity based on expert labels is learned and this reduces the quality of the work substantially.
A k-NN similarity graph is built using different p-distances (the authors find p=4 and k=30 work best) and the shortest path between two given images is found using Djikstra’s algorithm. When computing surrogate quality metrics and for determining the optimal path in general, an important problem (which is acknowledged in the paper) is the dependency of the geodesic distance on the length of the path. I think an obvious solution would have been a scalar bias, which reduces the dissimilarity for each extra intermediate image in a given path, which could have been found by cross-validation.
6. Constructive feedback
- It would have been interesting/necessary to discuss the recent Nature article "Dermatologist-level classification of skin cancer with deep neural networks" in this context. In this important work, a network of semantic similarity between different subgroups of skin lesions is derived following the work on fine-grained recognition.
Minor comment for abstract: "progressively visually skin lesions" should be "progressively visually similar skin lesions"
REVIEWER #3
1. Level of expertise
- Expert
2. Recommendation
- Borderline
3. Summary of the paper
- This paper addresses the problem of finding progressively similar skin lesion images. For example, given source, target and a set of images, the objective is to order the set (or subset) of images between source to target so that the order progressively maintains similarity. The proposed approach is to:
1) Create a simple graph whose edge weights are determined based on weighted cosine dissimilarity between the CNN feature vectors (different modalities)
2) Decide the connectivity of the graph based on kNN
3) Apply shortest path between source and target to find the intermediate order.
4. Strengths
- The problem in hand is interesting. The graph based approach is also interesting.
5. Shortcomings
- 1) Graph connectivity is crucial and there is no clear way of obtaining it properly. Cross validating k is not feasible for larger problems.
2) Deep Net is pertained on ImageNet dataset, which is very different from the images for which the feature vectors are obtained.
3) The approach, though not very trivial, looks pretty straight forward to me.
REVIEWER #4
1. Level of expertise
- Knowledgeable
2. Recommendation
- Borderline
3. Summary of the paper
- The paper proposes a way to compute geodesic paths in image space within a database of skin lesion images. Given a pair of images, a path consists of a set of images along the similarity manifold in image space.
4. Strengths
- Interesting application, and the paper is overall well written.
5. Shortcomings
- - The practical value is not entirely clear to me. How would a doctor choose an appropriate target image given an image of a patient. I think an application for predicting diagnostic labels using nearest neighbours seems more valuable.
- It's also not clear how the 'clinical' image is useful, as those seem to be less standardised than the dermoscopic ones and might show the lesion at very different and somewhat arbitrary scale
6. Constructive feedback
- I think the motivation for extracting visual paths needs to be made stronger. One application could involve prediction of lesion progression.